Data Analytics Course for Informed Financial Investing
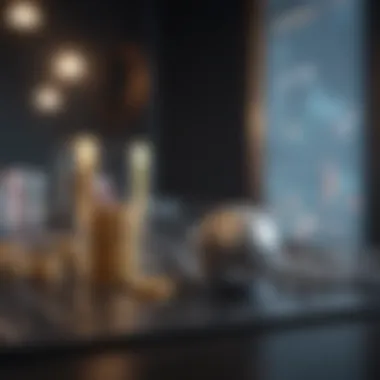
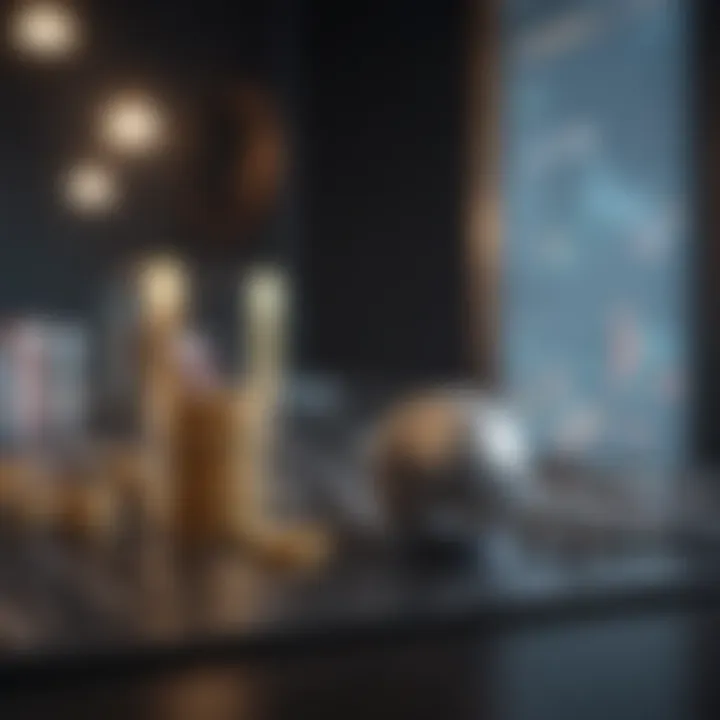
Intro
In today's fast-paced world of finance, understanding data analytics has become the cornerstone of making informed investment decisions. This is more than just a fancy tool for analysts; it’s a fundamental approach that every investor must grasp if they wish to navigate the complexities of the financial landscape effectively. The marriage of data and finance isn’t merely beneficial—it’s essential for those seeking to stay ahead.
The specialized course aiming at integrating data analytics into finance education is built upon key concepts that serve as the bedrock for informed investing. From basic financial terminology to sophisticated analytical techniques, each facet is meticulously designed to enrich the learner's experience.
Building a solid foundation in these core concepts not only enhances one's ability to analyze market trends but also equips investors with the skill set required to identify opportunities and mitigate risks. In this discussion, we’ll venture into the heart of what makes this course pivotal, examining not just the content but the very essence of data proficiency in finance.
Prolusion to Data Analytics in Finance
In today's financial ecosystem, the ability to interpret and leverage data analytics is not just useful; it's crucial. As market dynamics evolve and the volume of available data swells, professionals and investors alike must adapt to make informed choices. Data analytics acts as a lighthouse in the fog of vast information, guiding stakeholders toward sound investment decisions. Therefore, understanding this discipline becomes not just an academic exercise but a practical necessity for anyone looking to thrive in the financial sector.
Understanding Data Analytics
Data analytics is fundamentally about dissecting data sets to extract actionable insights. It's more than crunching numbers—it's about turning those numbers into narratives that inform strategy and decision-making. By employing various analytical methods, financial professionals can unveil patterns and trends that drive market behavior. A solid grasp of data analytics enables investors to recognize potential risks and opportunities faster than their competitors.
With tools like statistical analysis or machine learning frameworks like Python’s scikit-learn, individuals can mold raw data into comprehensible formats that facilitate strategic thinking. Concepts such as regression analysis or clustering can reveal correlations not initially apparent, adding depth to investment strategies.
Importance of Data Analytics in Finance
The importance of data analytics in finance can hardly be overstated. Here are several key points illustrating its significance:
- Risk Mitigation: Businesses can assess market volatility and identify potential downturns before they occur. This foresight helps in developing contingency plans.
- Enhanced Decision-Making: A data-driven approach leads to more informed decisions, enhancing the likelihood of achieving desired financial results.
- Portfolio Optimization: Investors can analyze asset performance over time, allowing for the fine-tuning of investment portfolios based on empirical evidence rather than guesswork.
- Customer Insights: Data analytics enables businesses to delve into customer behavior, tailoring products and marketing strategies that resonate with target audiences.
Businesses equipped with data analytics tools can turn the tide in competitive environments, transforming uncertainty into predictability.
In summation, data analytics paves the way for smarter investing, helping professionals flourish in the ever-evolving financial landscape. Understanding its principles lays the groundwork for success, making it an indispensable component of finance education.
Curriculum Overview of Data Analytics in Finance Course
A well-structured curriculum is like the backbone of any adept educational program, especially when it revolves around data analytics in finance. It shapes the pathway for students and professionals alike, guiding them through a pragmatic journey of acquiring essential skills and knowledge. This course offers a detailed delve into myriad facets of finance through the lens of data analytics. It is not just about crunching numbers; instead, it involves understanding interactions within complex data sets, developing critical thinking skills, and applying tools to make well-informed financial decisions.
The curriculum is purposely crafted to resonate with everyone from seasoned investors seeking to sharpen their acumen to novices eager for a head start. By emphasizing both fundamental and advanced analytical techniques, the program prepares participants to tackle real-world challenges they will inevitably encounter in the financial landscape.
Core Topics Covered
Statistical Analysis
Statistical analysis serves as the bedrock upon which many data-driven decisions stand. It encompasses methods to collect, review, analyze, and draw conclusions from data. This course dives deep into concepts like probability distributions, hypothesis testing, and regression analysis. These elements are critical as they provide insights into market trends and investor behavior.
A defining characteristic of statistical analysis is its ability to quantify uncertainty and risk. Considering modern investing's volatility, the capability to interpret data statistically has become invaluable. The course particularly highlights how statistical models can predict outcomes based on historical data, making it a beneficial addition to any investor's toolkit.
However, students must also consider its limitations. Statistical analysis relies heavily on the quality of data; poor quality data can lead to misguided conclusions. Balancing the strengths and drawbacks of this approach is crucial for meaningful financial insight.
Data Visualization Techniques
Data visualization techniques play an essential role in translating complex data into digestible formats. In an age flooded with information, visuals—graphs, diagrams, and charts—help distill insights that might otherwise be hidden in raw data. This component of the course focuses on best practices for creating impactful visual aids that tell a story, guiding investment decisions and strategies.
One key characteristic of data visualization is its ability to simplify information presentation to diverse audiences. It’s not merely about creating flashy graphics, but ensuring that critical data points are highlighted effectively. For aspiring finance professionals, mastering this skill is crucial since it fosters better communication with stakeholders.
Yet, a word of caution is warranted here: misleading or overly complex visualizations can distort perception, leading to uninformed decisions. Thus, comprehending the ethical responsibility behind data representation is an integral part of the training.
Machine Learning in Finance
The surge of machine learning in recent years has transformed how financial data can be analyzed and leveraged. This segment of the curriculum introduces concepts such as predictive modeling and algorithmic trading, equipping students with knowledge on how machines can automate and optimize investment strategies.
A prominent characteristic of machine learning is its capacity to sift through vast amounts of data at lightning speed. Unlike traditional methods, which require more human intervention, machine learning algorithms can learn from data patterns and improve over time—enhancing efficiency and accuracy in decision-making processes.
Nevertheless, reliance on machine learning also comes with its hurdles. It demands strong quantitative skills and can often feel like a black box to those unfamiliar with its mechanics. Hence, understanding its applications—along with its limitations—is pivotal for a balanced, informed approach.
Course Structure and Duration
The course structure is designed to cater to various learning preferences, combining theoretical knowledge with hands-on practice. Typically, it spans several weeks to a few months, allowing ample time for deep dives into each subject matter. Each module is carefully timed to ensure a comprehensive understanding of fundamental principles before moving on to more complex topics.
Enabling real-world application through projects, workshops, and case studies, the curriculum is crafted to ensure that participants emerge not just with knowledge but with practical expertise that can be applied directly within their organizations.
Ultimately, this curriculum stands as a robust framework guiding finance enthusiasts towards mastering data analytics, structuring their knowledge to foster informed investment decisions.
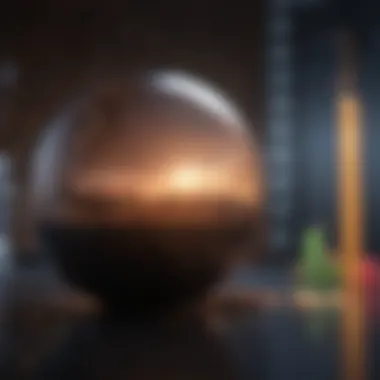
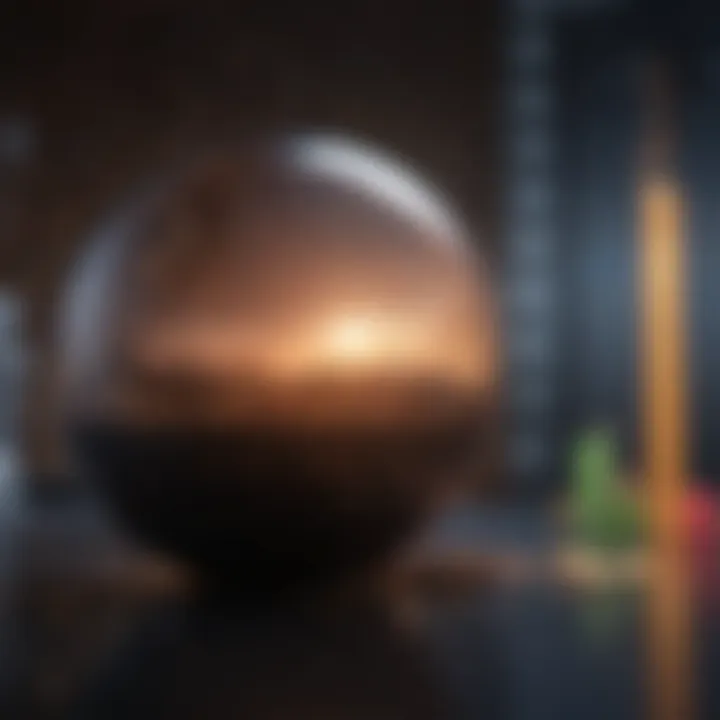
Skills Developed through Data Analytics
As we navigate the complexities of finance, the significance of data analytics can't be overstated. The realm of finance today demands more than just a gut instinct or traditional methods. Investors and professionals are increasingly leaning on data analytics to fine-tune their strategies and make decisions grounded in evidence rather than conjecture. Within a well-structured course on this subject, various skills are cultivated, all of which contribute to a robust understanding of markets and investments.
Analytical Thinking
Analytical thinking is one of the cornerstones of decision-making in finance. This skill involves breaking down complex problems into manageable parts, enabling learners to make sense of vast datasets. Through the course, participants learn to discern patterns and correlations that might not be immediately apparent. They are taught to ask the right questions and to verify assumptions with data-backed evidence.
Here are some elements that underline the importance of analytical thinking in finance:
- Problem-Solving: The ability to translate abstract financial theories into practical applications is key. Analytical thinking fosters this skill, allowing for effective resolution of financial challenges.
- Data Interpretation: Understanding what the data signifies can have a profound impact on investment choices. A background in analytical thinking means professionals can interpret trends or shifts in the market effectively.
- Strategic Planning: With strong analytical capabilities, finance professionals can formulate plans that are informed and nuanced. They can anticipate future financial scenarios based on historical data, adding robustness to strategic initiatives.
Technical Proficiency
Technical proficiency encompasses the necessary skills and knowledge of tools that one must wield in today's financial contexts. From programming languages to software tools, possessing technical skills ensures that investors and finance professionals can confidently navigate the intricacies of data analytics.
Programming Languages
Programming languages like Python and R have become inextricable from the field of data analytics. Python, in particular, stands out due to its sheer versatility and ease of learning. Here’s why it is favored:
- Ease of Use: Python’s syntax is clear and straightforward, making it an easy entry point for beginners, allowing investors to focus less on language hurdles and more on analytics.
- Robust Libraries: With libraries such as Pandas and NumPy, analysts can swiftly process large datasets and perform complex calculations, which can unearth insights without excessive manual work.
- Community Support: A rich ecosystem of community support means that users can find resources and assistance easily, fostering a continuous learning environment.
However, it also has its unique features that might be seen as drawbacks:
- Speed Issues: While it offers simplicity, Python may not perform as quickly as lower-level languages in some data-heavy scenarios, but for most financial tasks, it strikes a fine balance.
Software Tools
Tools like Tableau and Microsoft Power BI epitomize the need for effective data visualization in finance analytics. Understanding how to use such tools can catapult someone's analytical capabilities to another level. Here’s why these tools are essential:
- Visual Representation: Software like Tableau allows analysts to create compelling data stories that make insights more digestible for stakeholders. This visual engagement can drive better decision-making processes.
- Integration: Many of these tools can seamlessly integrate with other databases and software, creating a cohesive environment for analysis.
Nonetheless, they have certain aspects to watch out for:
- Cost Considerations: Advanced software packages may require significant investments, which can be daunting for smaller firms or individual investors.
- Learning Curve: Some professionals may encounter sticker shock when faced with the steep learning curve associated with advanced features, potentially limiting immediate usability.
Methodologies in Data Analytics
Understanding the methodologies behind data analytics is paramount, especially in a realm as dynamic as finance. These methodologies serve as the backbone for how data is interpreted and applied to harness insights that fuel investment strategies. By embracing different analytical methods, financial professionals can not only improve their decision-making but also stay a step ahead in an ever-competitive field.
Descriptive Analytics
Descriptive analytics is the bedrock of data analytics. This technique focuses on summarizing historical data to understand trends and outcomes. By employing various statistical measures, descriptive analytics offers a clearer view into what has happened, often leading to insights that are pivotal for future decisions.
In finance, descriptive analytics can manifest through dashboards that represent key performance indicators, such as revenue growth or customer acquisition rates. This can help stakeholders draw connections and understand patterns, leading to more informed choices.
"Without understanding what has transpired, predicting the future becomes a shot in the dark."
Some common techniques utilized in descriptive analytics include:
- Data Aggregation: Compiling data from various sources to create comprehensive reports.
- Basic Statistical Tools: Utilizing means, medians, and modes to summarize data sets.
- Data Visualization: Crafting visual interpretations, like graphs and charts, to present findings clearly and effectively.
The end goal here is to set a solid foundation upon which further analyses, and ultimately, predictive and prescriptive analytics can build.
Predictive Analytics
Predictive analytics takes the analysis a step further by leveraging historical data to forecast future outcomes. This methodology utilizes statistical algorithms and machine learning techniques to identify patterns and predict trends. In finance, becoming adept at predictive analytics is crucial as it allows investors to anticipate market fluctuations and possible investment opportunities.
For instance, an investor might use predictive analytics to analyze stock performance by inputting variables like previous prices, volume, and market conditions to calculate future worth. Such insights allow for better strategic planning and risk management.
Methodologies in predictive analytics often include tools and concepts like:
- Regression Analysis: Identifying relationships between variables and predicting outcomes based on these correlations.
- Time Series Analysis: Examining data points collected or recorded at specific time intervals to forecast future events.
- Machine Learning Algorithms: Implementing models that learn from data to improve predictions over time.
In a nutshell, predictive analytics transforms data into foresight, empowering investors with valuable knowledge to navigate the financescape.
Prescriptive Analytics
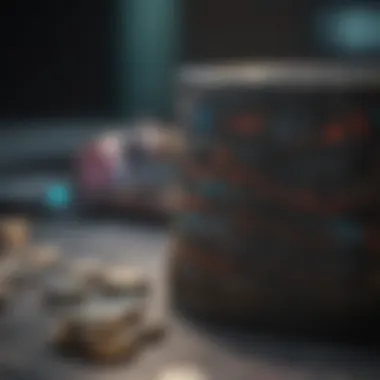
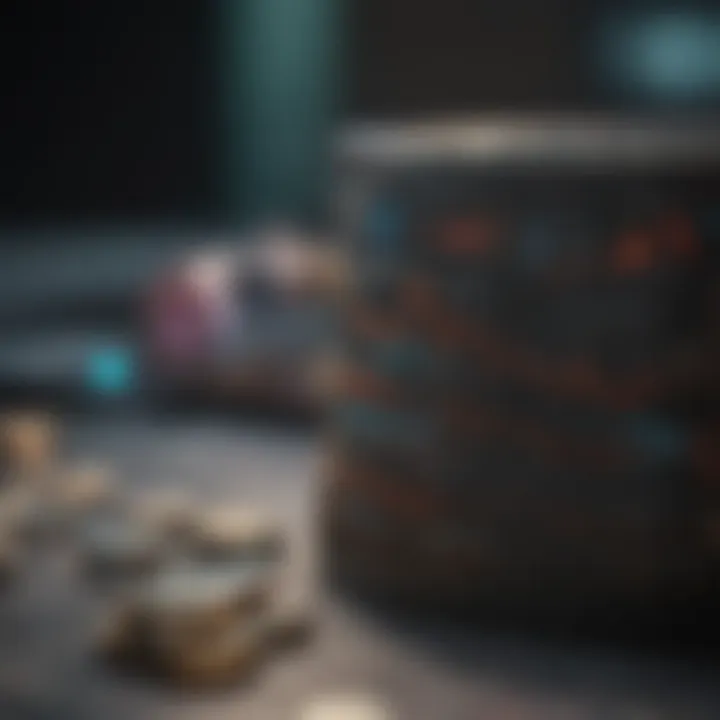
As the final layer of analytics, prescriptive analytics provides recommendations based on data-driven insights. This methodology not only anticipates outcomes but also suggests actions to achieve desired results. Financial professionals leverage prescriptive analytics to optimize decision-making processes and enhance profitability.
For example, a financial advisor might employ prescriptive analytics to determine the best investment strategy based on individual risk tolerance and market conditions. This method enables tailored advice that aligns closely with clients’ goals.
Prescriptive analytics typically employs:
- Optimization Models: Techniques that help in identifying the best possible solution among various options while taking constraints into account.
- Simulation: Running various scenarios to assess potential outcomes based on different actions.
- Decision Analysis: Utilizing frameworks to understand the implications of different financial decisions.
Practical Applications of Data Analytics in Finance
In today's fast-paced financial world, the application of data analytics is not just a nice-to-have; it's essential. This section delves into the significant ways data analytics shapes the finance landscape. By examining specific applications, professionals can make better, more informed decisions.
Risk Assessment and Management
Risk assessment is a cornerstone of finance, and it is here that data analytics truly shines. It enables investors to evaluate, and subsequently mitigate, risks associated with their portfolios. Using advanced statistical methods, firms can quantify risks in ways that inform investment strategies.
- Predictive Models: Financial analysts harness predictive modeling to foresee potential market downturns. For instance, employing algorithms that analyze historical market data helps in understanding patterns that may signal future volatility.
- Scenario Analysis: Scenario analysis allows firms to envision how their investments might behave under different circumstances. This could involve stress-testing a portfolio against extreme market conditions, thereby preparing stakeholders for possible negative impacts.
Ultimately, strong risk management fuels investor confidence, allowing them to make bolder decisions with a clearer understanding of potential setbacks.
Investment Performance Analysis
Measuring investment performance is another critical area where data analytics plays an influential role. By systematically analyzing returns relative to risks, investors can determine if their strategies are yielding desired outcomes.
- Performance Metrics: Tools like the Sharpe ratio or alpha metrics enable analysts to assess risk-adjusted returns. These metrics provide insights into how well an investment compensates for the risk taken.
- Benchmarking: Comparative analysis against market benchmarks helps investors gauge whether their portfolios are outperforming or underperforming industry standards. This information is invaluable, as it can inform adjustments to an investment strategy based on empirical data rather than hunches.
Through straightforward performance analysis, investors gain a nuanced understanding of their investments, allowing them to refine or pivot their strategies effectively.
Customer Insights and Retention
In the finance sector, understanding your customer base can differentiate success from struggle. Data analytics can help firms dive deep into customer behaviors and preferences, enhancing retention strategies.
- Segmentation Analysis: By segmenting customers according to behavior, preferences, or demographic traits, companies can tailor their offerings to meet specific needs. This could involve recognizing patterns where certain customer segments may be likely to churn.
- Predictive Retention Strategies: Employing predictive analytics enables firms to identify customers at risk of leaving and proactively address potential concerns. For instance, if historical data shows that clients who frequently contact support are more likely to leave, a company can step up its customer service efforts.
Understanding customers through a data lens is not just about making sales; it’s about fostering long-lasting relationships that enhance loyalty and satisfaction.
"In the world of finance, insights derived from data analytics are no longer optional but fundamental for sustained success."
Thus, the practical applications of data analytics transcend mere number-crunching. They empower financial professionals by providing tools and insights necessary for sound decision-making in an ever-evolving financial landscape.
Case Studies Demonstrating Data Analytics Success
Understanding how data analytics transforms financial decision-making is best approached through concrete examples. Case studies illustrate the real-world applications and advantages of data analytics in finance. They showcase how businesses and investors apply analytical methods to achieve results, making the significance of this subject truly come alive. This examination is not merely academic; it's about understanding the tangible benefits that powered decisions have on investments and risk management.
Real-World Examples
In recent years, several companies have harnessed the power of data analytics to optimize their strategies effectively. Let's discuss a few standout cases:
1. Goldman Sachs:
Goldman Sachs embraced machine learning models to analyze vast amounts of trading data. By leveraging these models, they improved their trading algorithms, which led to more accurate predictions of market movements. The enhanced processing power enabled them to sift through data at remarkable speeds, translating to more informed decisions and increased profitability.
2. American Express:
In an effort to understand customer behavior, American Express utilized predictive analytics to identify potential churn. By analyzing transaction patterns and customer feedback, they created targeted marketing campaigns tailored for customer retention. Notably, this approach resulted in reducing churn rates significantly and improving customer loyalty.
3. JP Morgan:
JP Morgan applied natural language processing (NLP) to analyze unstructured data from financial reports, news articles, and market sentiment. This revolutionary approach has been pivotal in getting insights about public sentiment surrounding companies, leading to better-informed investment decisions. They have reported improved compliance and operational efficiency as a result.
The implications of these cases go far beyond mere numbers. They reflect strategic foresight, risk management, and business sustainability. Investors today must recognize the imperative of integrating analytics into their processes.
Lessons Learned
The journey through these case studies brings several key insights that stand as pillars of effective analytics in finance:
- Data Quality is Paramount:
Poor data will lead to misleading conclusions. The companies that succeeded were those that prioritized data integrity. Ensuring that the data collected is accurate and relevant is the foundation upon which successful analytics is built. - Adaptability is Crucial:
The financial landscape is always evolving. Organizations that remain flexible, adapting to new technologies and embracing evolving analytical tools frequently stay ahead of the curve. It’s about learning from previous experiences and being willing to change as needed. - Collaboration is Essential:
Data analytics isn't solely the domain of IT departments; it's a collaborative effort. Engagement between various departments enhances insight generation and promotes a comprehensive understanding of data. It’s essential for everyone involved, from analysts to senior management, to communicate openly about findings and strategies. - Continuous Learning:
The field of data analytics is always advancing. Constantly improving skills and knowledge relevant to data tools and methodologies, ensures that financial professionals remain competitive.
Embracing data analytics isn't just about crunching numbers; it’s about fostering a culture of data-driven decision-making that ultimately leads to better investing outcomes.
In summary, the case studies not only highlight successful implementations but also serve as vital lessons for those looking to navigate the complex waters of finance using data analytics. Recognizing these factors can empower individual investors and financial professionals to make informed, strategic decisions that shape their future endeavors.
Challenges in Implementing Data Analytics
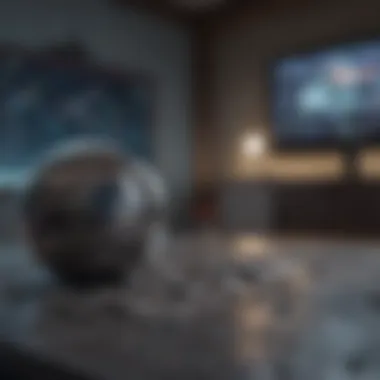
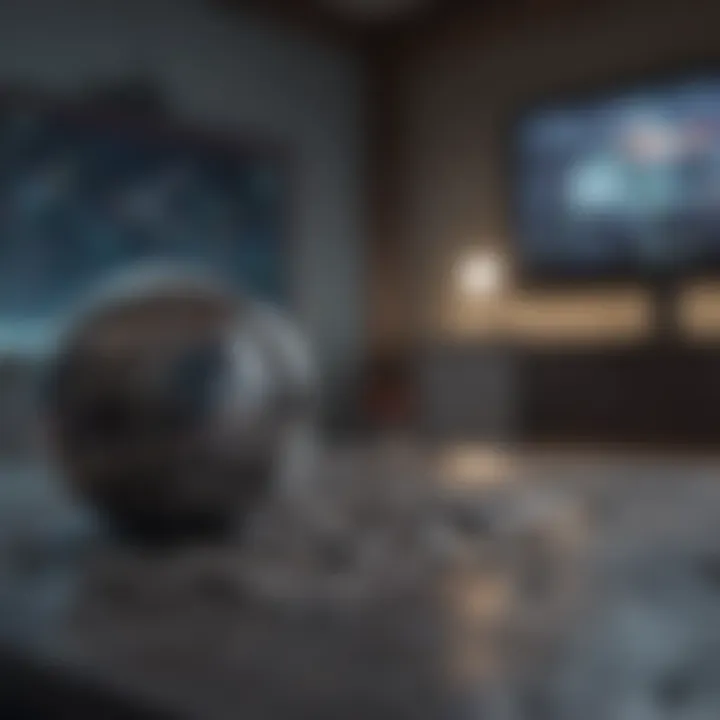
The integration of data analytics in finance, while promising, does not come without its set of challenges. As we venture deeper into this domain, it is crucial to acknowledge these obstacles, as they can hinder the utility and effectiveness of data-driven strategies. Understanding these challenges enables financial institutions and individual investors to foster a conducive environment for analytics to thrive. In this section, we will shine a light on two significant challenges: data quality issues and resistance to change.
Data Quality Issues
The cornerstone of any successful data analysis effort is the quality of the data being utilized. Unfortunately, many organizations often find themselves grappling with inaccurate or incomplete data. Consider this: if a chef is provided with spoiled ingredients, no matter how skilled they are, the dish will not meet expectations. Similarly, when financial analysts work with poor quality data, their insights can become misleading, undermining the very purpose of data analytics.
Poor data quality can stem from various sources, including:
- Data Entry Errors: Human mistakes during the entry process can lead to erroneous information.
- Inconsistent Formats: Variations in data formats can create discrepancies, complicating analysis.
- Outdated Data Sets: Using old data can result in analyses that do not reflect current market realities.
To mitigate these issues, organizations need to implement robust data governance frameworks. Such frameworks focus on establishing clear protocols for data collection, storage, and processing. Regular data audits can help identify and rectify problems before they escalate. In summary, ensuring high-quality data is essential for producing reliable analytics that can inform investment strategies and decision-making.
Resistance to Change
While the advent of analytics tools presents various advantages, the road to adoption isn't always smooth. Resistance to change is a common barrier that organizations encounter when integrating data analytics into existing frameworks. Employees might worry that automation could replace their roles or may simply be comfortable with traditional methods that they have always used.
This resistance can manifest itself in multiple ways:
- Skepticism: Some team members may doubt the utility or accuracy of analytics.
- Fear of Job Security: Others might feel threatened by the technology, fearing it could render their skills obsolete.
- Cultural Barriers: A company culture resistant to innovation may slow down the adoption of new processes.
Overcoming resistance requires strong leadership and effective communication. Organizations should provide training to help employees understand not just how to use the tools, but why they are important. Highlighting success stories and demonstrating the tangible benefits of data analytics can also play a pivotal role in shifting perspectives.
"When it comes to implementing new strategies like data analytics, the old saying rings true: 'Out with the old, in with the new.' But getting there requires more than just knocking on doors of data; it demands a shift in mindset."
Future Landscape of Finance Education
In the ever-evolving realm of finance, the landscape of education is undergoing significant transformation, driven largely by technological advancements. As financial professionals and individual investors look to enhance their expertise, the focus on incorporating data analytics into finance courses becomes increasingly critical. New methodologies and technologies are reshaping how finance is taught and understood, fostering an environment that promotes informed decision-making.
Emerging trends such as blockchain technology and artificial intelligence are carving out new pathways for learning and application in finance. These advancements not only provide fresh insights but also equip learners with the necessary tools to tackle the complexities of today's financial ecosystem. The integration of such technologies into the curriculum is transforming traditional learning models into a more dynamic and responsive framework.
"Education must keep pace with the rapidly changing financial landscape; otherwise, it risks becoming obsolete."
Emerging Technologies
Blockchain
Blockchain technology stands at the forefront of finance innovation. It is prized for its ability to offer transparency and security in transactions, ensuring that financial data is recorded in a tamper-proof manner. This characteristic makes it a beneficial choice for finance courses. By understanding blockchain, students grasp how this decentralized ledger system can prevent fraud and streamline processes.
One of the unique features of blockchain is its immutability; once a record is added, it cannot be altered. This allows for a clear audit trail, which is invaluable for regulatory compliance—an essential aspect of finance education. However, while blockchain holds numerous advantages, such as increasing trust among stakeholders, it is worth noting that scalability issues and the energy consumption involved in some blockchain networks can be pertinent discussions in educational settings.
Artificial Intelligence
When one speaks of artificial intelligence in finance, its impact is hard to overlook. AI enhances data analysis by using algorithms that can process vast datasets more efficiently than any human ever could. This functionality allows for deeper insights and supports predictive analytics in investment strategies. Consequently, AI-enhanced tools are invaluable in finance education, as future professionals learn to leverage them for forecasting market trends.
A distinctive feature of artificial intelligence is its capability to adapt and learn from new data. This adaptive learning enables tools to improve their accuracy over time. However, while AI offers significant advantages like speed and precision, concerns about algorithmic bias and the potential for misuse in financial decision-making are essential considerations that must be addressed in the curriculum.
Evolving Educational Tactics
As the financial industry evolves, so too must the tactics employed in education. Traditional lecture-based methods are giving way to more interactive and experiential learning approaches. Students are now engaging in case studies, simulations, and practical applications that mirror real-world scenarios. Such tactical shifts not only make learning more engaging but also prepare students for the unpredictable nature of the financial market.
Collaboration is key; group projects and teamwork enhance the learning experience by reflecting the collaborative nature of the finance industry. This adaptability in educational tactics ensures that students are not only learning theory but also applying it in ways that mirror actual financial practices. In summary, the future of finance education is bright, driven by innovative technologies and teaching methods that foster a deeper understanding of data analytics.
Epilogue
In wrapping up our exploration of data analytics within finance education, it becomes abundantly clear how vital this intersection is, not only for current industry players but also for aspiring investors navigating the complexities of the financial landscape. The course outlined herein serves as a cornerstone for fostering essential skills, understanding methodologies, and applying knowledge to real-world scenarios.
Summing Up the Course's Impact
To put it plainly, the impact of a data analytics course in finance is multifaceted. First and foremost, it equips participants with the ability to analyze vast amounts of data effectively—turning what may seem like a mountain of figures into meaningful insights. Graduates emerge with a solid grounding in statistical techniques and analytical tools necessary for making informed decisions.
A key takeaway from this course is its focus on practical applications, enabling learners to bridge theory and practice. Engaging case studies reinforce the lessons taught, showcasing tangible results in risk assessment, customer insights, and even investment performance monitoring. This hands-on experience instills confidence, allowing investors to navigate uncertainties in the market with greater assurance.
"Harnessing data analytics is like having a compass on a foggy day; it guides you through the complexities and helps avoid pitfalls."
In addition, the course prompts learners to consider the ethical implications of analytics in finance, advocating for responsible decision-making grounded in data transparency. A well-rounded investor is not just someone who understands numbers but also one who recognizes the broader impact of their decisions.
Encouragement for Continuous Learning
The world of finance, much like the ocean, is vast and constantly changing. To remain relevant and competitive, continuous learning is not just a suggestion; it’s a necessity. Whether through formal education or self-directed study, there is always an opportunity to deepen one’s understanding of evolving data analytics techniques.
Investors should actively seek out avenues to expand their knowledge. This can include participating in workshops, engaging with online forums, and subscribing to industry publications. Platforms like Reddit and specialized finance groups on Facebook can be rich resources for discussions and insights among peers. Moreover, leveraging online courses for advanced topics—like machine learning or blockchain—can be incredibly beneficial.
In sum, while the foundational course provides a robust starting point, the journey does not end there. As markets evolve and new tools emerge, so too must the skills of those who seek to invest wisely. Only through ongoing education can individuals truly harness the power of data analytics, ensuring that their investment strategies remain informed and proactive.