Key Skills for Effective Data Analysis
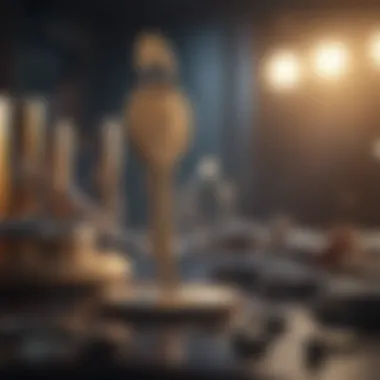
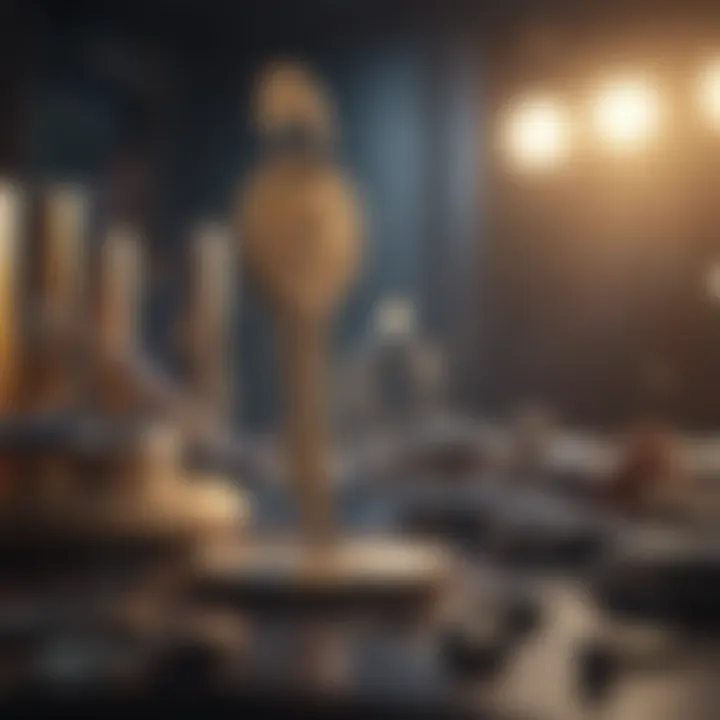
Intro
In today's digital age, data is lurking almost everywhere — from social media posts to online transactions. While numbers can be fascinating, the real magic lies in understanding what those numbers say and how they guide decisions across all sectors. This article breaks down the essence of data analysis skills. It walks you through crucial concepts, relevant tools, and real-world applications.
Effective data analysis goes beyond crunching numbers. It encompasses the ability to interpret, visualize, and communicate findings in a way that drives action. The significance of these skills is gaining major traction as organizations increasingly rely on data-driven insights for strategic planning.
Key Concepts and Definitions
Data analysis skills can be quite multifaceted, but let's lay the groundwork by defining some popular terms.
- Data Literacy: This is the ability to read, understand, create, and communicate data as information. In simple terms, if someone can interpret a graph or sales report effectively, they possess a level of data literacy.
- Statistical Analysis: Often deemed the backbone of data analysis, wrapping one’s head around statistical techniques equips analysts with the tools to draw conclusions.
- Data Visualization: Creating visual representations of data isn’t just to make it pretty; it enhances understanding and recognition of patterns that might be hidden in raw numbers.
Navigating these concepts sets the stage for further exploration. Let's discuss why grasping these terms is crucial for anyone in finance or investment.
Significance of Understanding Data Analysis Skills
Understanding data analysis skills isn’t merely a voyeuristic endeavor. It’s akin to holding a magnifying glass to your decision-making process. In the world of finance, these skills empower investors to make informed, multidimensional evaluations before staking their money.
Often, in financial discussions, one might hear phrases like "the market is reacting to this data point" or "the underlying trends suggest" Without the right skills, such statements may just float by like autumn leaves. Consequently, being data-savvy means optimizing opportunities while avoiding pitfalls that slip through the cracks when insights are disregarded.
Moreover, as the data landscape continues to evolve, possessing these skills is rapidly transitioning from a nice-to-have into a must-have. Failing to stay ahead of the curve may lead to suboptimal investment strategies, missed opportunities, or irrelevant conclusions.
"Data is a precious thing and will last longer than the systems themselves." - Tim Berners-Lee
With these foundational concepts in mind, we'll dive deeper into practical applications in various fields, such as finance and research. Be prepared to explore how these skills translate into measurable outcomes.
Understanding Data Analysis Skills
In an era where decisions are increasingly driven by data, the understanding of data analysis skills has become not just beneficial but essential. Individuals and organizations that grasp these skills are better equipped to navigate complex information landscapes. This understanding opens the door to a world of insights that are often overlooked by those who operate solely on intuition or past experiences.
Effective data analysis skills encompass a mix of technical know-how and critical thinking capabilities. It’s not just about knowing how to use software to manipulate numbers; it’s about interpreting what those numbers mean in practical terms. A keen analyst can transform raw datasets into actionable insights, guiding strategic decisions across various sectors like finance, marketing, healthcare, and beyond.
Furthermore, incorporating analyses into everyday business practices can significantly enhance productivity and cost-effectiveness. For instance, a small business that regularly reviews sales trends and customer behaviors can better tailor its offerings, ultimately boosting customer satisfaction and loyalty. A solid grasp of data analysis skills enables these organizations to pivot quickly in response to market changes.
"Data is a precious thing and will last longer than the systems themselves." – Tim Berners-Lee
What stands out about understanding data analysis is its applicability across diverse contexts. Whether it's a financial investor examining stock performance trends or a healthcare professional analyzing patient care patterns, the core skills required remain similar. Furthermore, as technology advances, new tools emerge that help simplify the data analysis process, making these skills even more crucial.
In sum, understanding data analysis skills not only enhances one's capability to make informed decisions but also fosters a deeper comprehension of underlying trends, risks, and opportunities. Without a doubt, these competencies are indispensable for anyone looking to thrive in today's data-rich environment.
Defining Data Analysis Skills
Data analysis skills refer to the capabilities required to collect, process, and analyze data effectively. These skills vary widely, encompassing a range of techniques that help in transforming data into meaningful insights. At its core, data analysis involves the ability to discern patterns and trends that could inform diverse business strategies. Each component—statistical knowledge, data manipulation, analytical thinking, and technical proficiency—plays a vital role in this definition.
To break it down further:
- Statistical Knowledge: Understanding how to describe and infer data through statistical methods. This includes being familiar with both descriptive and inferential statistics.
- Data Manipulation Techniques: Mastery of cleaning, transforming, and integrating data sources is crucial to ensure accuracy in analysis.
- Analytical Thinking: Critical and logical reasoning skills that enable analysts to solve problems creatively and effectively.
- Technical Proficiency: Knowledge of the software and programming languages essential for conducting analyses, including proficiency in tools like Excel, R, or Python.
Ultimately, possessing a solid foundation in these areas equips individuals to conduct thorough analyses, leading to more informed decision-making.
Importance of Data Analysis in Modern Contexts
The role of data analysis in the modern world cannot be overstated. As industries become more data-driven, the ability to analyze information has transcended beyond mere data interpretation—it's now a strategic necessity.
In business settings, a robust data analysis process informs marketing strategies, enhances customer experience, and improves operational efficiencies. Companies that leverage data often experience an increase in revenue due to well-informed decision-making. For example, consider a retail firm that employs data analysis to understand purchasing trends among its customers. By doing so, it can better manage inventory, optimize pricing, and launch targeted marketing campaigns.
Moreover, in the realm of finance, data analysis is critical for risk assessment and portfolio management. Investors relying on historical data trends to guide their investment strategies can avoid substantial pitfalls.
Additionally, with the ongoing rise of Big Data, organizations that fail to harness data analysis skills find themselves at a significant disadvantage.
In summary, understanding and implementing data analysis is vital for anyone in today’s job market. The ability to navigate data not only aids in making wise choices but also opens doors to innovation and long-term success.
Core Components of Data Analysis Skills
The field of data analysis is a vast and ever-evolving terrain. For those looking to navigate this landscape, understanding the core components of data analysis skills becomes vital. These components serve as the building blocks that enable individuals to engage in effective data analysis. Each element is interlinked, and mastery over them fosters precision in decision making, elevating insights derived from data.
Acquiring a sound grasp on these skill sets doesn’t just add weight to your resume; it lays the groundwork for thoughtful interpretation of data, ensuring that any conclusions drawn are based on solid underpinnings. Whether you’re diving into business analytics or scientific research, the significance of honing these skills is indisputable.
Statistical Knowledge
Statistical knowledge is the backbone of any data analyst’s toolkit. It provides the analytical framework necessary for interpreting data patterns and drawing reliable conclusions. Understanding its various dimensions, including descriptive statistics, inferential statistics, and probability theory, paves the way for employing data effectively in real-world scenarios.
Descriptive Statistics
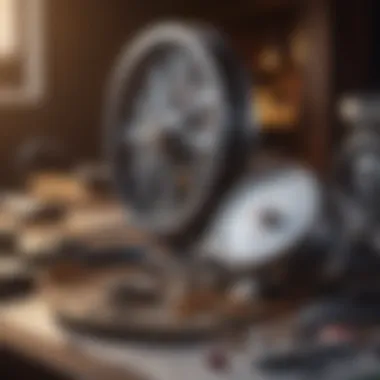
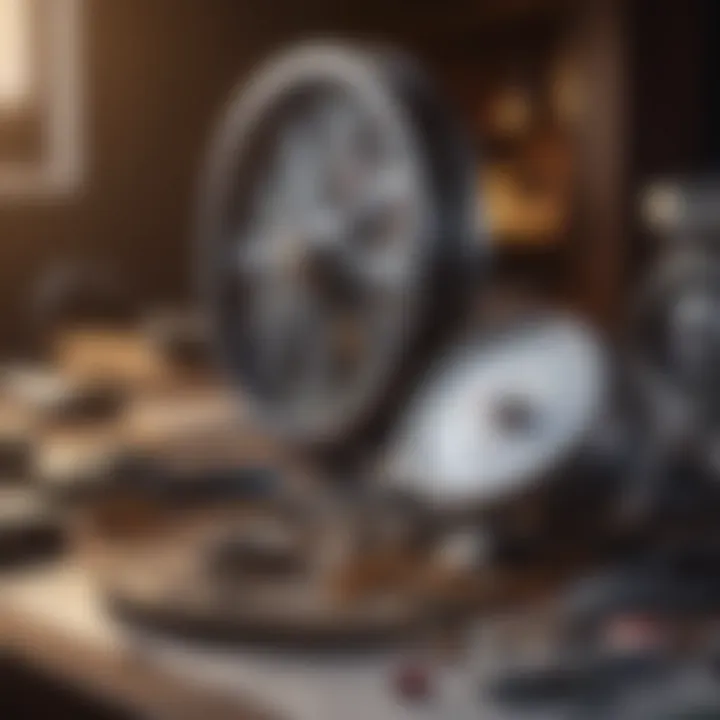
Descriptive statistics encapsulate the essential features of a dataset, offering a summary that’s intuitive for any stakeholder. This aspect is pivotal for providing a clear view of the data’s main characteristics, such as central tendencies and variability.
- Key Characteristic: At its core, this type of statistics utilizes measures like mean, median, and mode to simplify complex data sets.
- Benefits for This Article: Such summarization is crucial when presenting findings to audiences that may not be numerically inclined. Simplifying the data makes insights more digestible.
- Unique Features: The unique feature is that it allows for quick assessments, which can be particularly advantageous in fast-paced environments where decisions must be made swiftly.
Inferential Statistics
Inferential statistics extends beyond mere observation; it allows analysts to make predictions and generalizations about a population based on sample data. It bridges the gap between mere description and actionable insights, making it an invaluable part of any analytical effort.
- Key Characteristic: It often employs hypothesis testing, enabling analysts to make educated guesses about broader trends based on limited data.
- Benefits for This Article: This is especially useful when working with large data sets where surveying every single data point isn’t feasible.
- Unique Features: One of the disadvantages, however, is that it relies heavily on the quality of sample data; poor sampling can lead to misleading results.
Probability Theory
Probability theory forms the theoretical foundation of how likely outcomes are, thus guiding analysts in making sense of data phenomena. It plays a pivotal role in both descriptive and inferential statistics.
- Key Characteristic: It deals with the likelihood of an event occurring and helps quantify uncertainty in data analysis.
- Benefits for This Article: Understanding probability allows analysts to frame their observations within a risk context, essential for making informed decisions.
- Unique Features: However, probability can be daunting for some, as it often requires a solid understanding of mathematical principles.
Data Manipulation Techniques
Once data has been collected, the next step is manipulation. This stage can drastically affect the quality of insights that can be drawn. Effective data manipulation techniques such as data cleaning, transformation, and integration are each essential in producing reliable results.
Data Cleaning
Data cleaning addresses the inconsistencies and inaccuracies in datasets. That’s where the hard work starts, and it’s often the most time-consuming step in analysis.
- Key Characteristic: This process involves identifying missing values, duplicates, and errors that may skew results.
- Benefits for This Article: Clean data ensures that conclusions drawn are valid, fostering trust in the results.
- Unique Features: While being meticulous is beneficial, the downside is the potential for overlooked errors, which may arise from fatigue or oversight.
Data Transformation
Data transformation modifies the format, structure, or values of data to make it suitable for analysis. It’s about taking raw data and reshaping it to better fit analytical requirements.
- Key Characteristic: Operations may include normalizing data ranges and aggregating data to focus on broader trends.
- Benefits for This Article: Transforming data can aid in revealing patterns that raw data may obscure, presenting a clearer picture.
- Unique Features: The risk here is that transformation may lead to loss of information if not handled carefully.
Data Integration
Data integration refers to combining data from different sources into a unified view. This practice is increasingly relevant, given the diverse channels through which data flows.
- Key Characteristic: Integrated data allows for a more comprehensive analysis by linking different sets of information together.
- Benefits for This Article: This leads to richer insights, enhancing the overall analytical narrative.
- Unique Features: However, integrating disparate data sources can introduce complexities and challenges in ensuring data compatibility.
Analytical Thinking
Analytical thinking is the lens through which data is interpreted. It encapsulates skills such as critical thinking, problem solving, and logical reasoning, all of which elevate the data analyst’s capabilities.
Critical Thinking
Critical thinking involves evaluating data with a discerning eye, promoting an approach where assumptions are regularly questioned.
- Key Characteristic: It emphasizes the importance of not taking data at face value, considering various aspects and angles.
- Benefits for This Article: This vigilance ensures that interpretations made are both valid and reliable, not merely parroting the data as it appears.
- Unique Features: Yet, excessive skepticism can sometimes stifle actionable insights due to over-analysis.
Problem Solving
The ability to solve problems is intrinsic to data analysis. This entails identifying gaps or issues within datasets and determining how to address them.
- Key Characteristic: It’s about strategically navigating obstacles that may block effective data interpretation.
- Benefits for This Article: Strong problem-solving abilities can lead to innovative analytical methods and unique insights, enhancing value.
- Unique Features: The downside can be a potential over-reliance on familiar solutions, which might inhibit creativity.
Logical Reasoning
Logical reasoning is the framework that helps analysts draw connections between data points and derive conclusions.
- Key Characteristic: It involves a methodical approach to making inferences from the data.
- Benefits for This Article: Being logically sound bolsters the credibility of findings and arguments presented.
- Unique Features: However, reliance on logic without considering contextual or qualitative factors can result in a narrow view.
Technical Proficiency
As the world digs deeper into the vast ocean of data, technical proficiency becomes essential. Familiarity with analytical tools, data visualization software, and relevant programming languages ensures that analysts can translate raw data into meaningful narratives.
Familiarity with Analytical Tools
Familiarity with analytical tools caters to the technical demand of the data analysis role. From spreadsheets to advanced statistical software, tool knowledge is pivotal for efficient analysis.
- Key Characteristic: Proficiency allows for automated processes, saving time and increasing productivity.
- Benefits for This Article: This enables analysts to enhance their skills and focus on value-adding activities rather than repetitive tasks.
- Unique Features: Lack of familiarity with essential tools can set back analytical effectiveness, leading to frustration.
Data Visualization Software
Data visualization software transforms raw data into visual formats, making it easier to interpret complex datasets. Tools like Tableau or Power BI excel at this.
- Key Characteristic: They enhance comprehension by translating numbers into graphical representations.
- Benefits for This Article: Effective visualizations often reveal patterns or trends that might go unnoticed in raw numbers.
- Unique Features: However, heavy reliance on visuals can lead to misinterpretation if they are not designed thoughtfully.
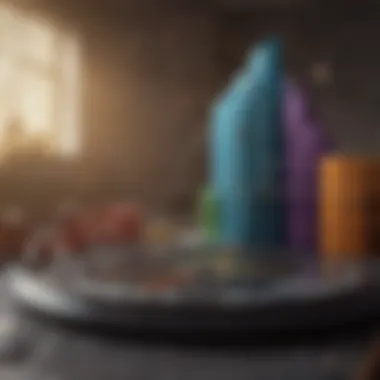
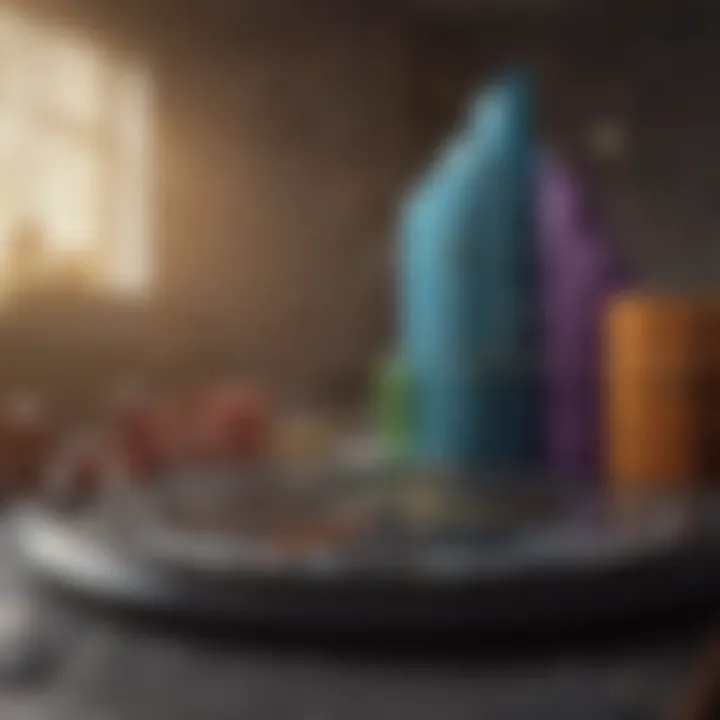
Programming Languages Relevant to Data Analysis
Knowledge of programming languages such as Python or R is indispensable for data analysts, providing them with the tools to manipulate and analyze data flexibly.
- Key Characteristic: Programming languages allow for writing custom scripts or using libraries designed for specific analytical tasks.
- Benefits for This Article: This adaptability pushes the boundaries of traditional analysis, offering deeper insights.
- Unique Features: That said, the learning curve can be steep for those without a technical background, creating barriers to entry.
Methodologies of Data Analysis
Understanding the methodologies behind data analysis is pivotal in today’s data-centric world. The right method can drastically affect the outcome of your findings and interpretations. Choosing the right approach helps analysts uncover critical insights that drive informed decisions in various sectors, including business and scientific research. Data methodologies serve as a framework, guiding the analyst through the often complex terrain of data interpretation while ensuring that the chosen approach aligns with specific objectives and requirements.
In this segment, we will delve into both qualitative and quantitative analysis methodologies, laying out how each can be applied to extract value from data. By probing into the nuanced elements, benefits, and considerations of these methodologies, a well-rounded comprehension is achieved, allowing practitioners and enthusiasts to adapt and implement skills adaptable across different fields, thus enhancing the relevance of data analysis in diverse applications.
Qualitative Analysis Methods
Qualitative analysis methods focus on understanding the deeper nuances and intangible qualities of data. Instead of just numbers, these methods seek to interpret the underlying meanings, experiences, and emotions behind the data, making it invaluable for creating rich, contextual insights.
Interviews and Focus Groups
Interviews and focus groups stand out among qualitative strategies for how they encourage open dialogue and interaction. An interview is a one-on-one conversation that seeks in-depth information from a participant. In contrast, focus groups gather a diverse group to discuss specific topics, which can yield a variety of perspectives. This method is particularly beneficial in market research, where understanding consumer sentiments is crucial.
The key characteristic of interviews lies in their structured or unstructured approach, allowing the interviewer to adapt questions based on responses. This flexibility promotes a deep dive into participant insights. Focus groups, on the other hand, harness group dynamics, which can spark conversations leading to unexpected insights.
However, the drawback is potential bias. An outspoken participant in a focus group might overshadow quieter ones, skewing the results. In interviews, the interviewer must be careful to avoid leading questions that could influence responses. Still, both methods offer the unique advantage of personal storytelling, revealing nuances often missed in quantitative analysis.
Content Analysis
Content analysis is another significant qualitative method, involving systematic coding and interpretation of textual, visual, or audio data to identify patterns or themes. This method is vital in media studies, cultural research, and any area involving extensive content review.
Its primary characteristic is that it offers a clear structure for analysis—researchers can categorize content according to predetermined themes. This method is highly beneficial when looking to understand trends or societal attitudes reflected through various channels such as social media or journalism.
Unique to content analysis is its ability to quantify qualitative data. For instance, counting how many times a specific theme appears in a body of texts provides a quantifiable measure of how prevalent that idea is in public discourse. However, analyzing context can be complex and time-consuming, requiring careful groundwork to ensure that the results are meaningful and not drawn from arbitrary patterns.
Case Studies
Case studies provide a deep, contextual exploration of a specific subject, such as a person, organization, event, or phenomenon. They offer a detailed view, integrating qualitative and quantitative data to understand the complexities of real-world situations. Their strength lies in providing comprehensive insights, allowing researchers to explore the specific conditions under which phenomena occur.
The key characteristic of case studies is their depth of analysis. Unlike other methods that offer a broader overview, case studies dive deep into specifics, often revealing intricate details and interconnections that are pivotal for understanding larger trends. This is why they are indispensable in both academic research and practical applications, enabling organizations or researchers to evaluate the effectiveness of certain strategies or approaches.
However, case studies can be time-consuming and may lack generalizability. What works for one case might not hold for another, so findings must be viewed within their context. Yet, their unique strength lies in narrative exploration, allowing storytelling that engages stakeholders and fosters understanding beyond mere statistics.
Quantitative Analysis Methods
Quantitative methods emphasize numerical data and statistical analysis, providing a framework to investigate relationships, patterns, and variances. These methodologies are essential for producing results that are replicable and objective, laying down a firm foundation for data-driven decision-making.
Survey Data Analysis
Survey data analysis involves gathering and quantifying large sets of responses to structured questions. It is a prevalent method for assessing public opinion, customer satisfaction, and market trends. The hallmark of this method is that it allows analysts to gather data from a significant population in a cost-effective and efficient manner.
Surveys can reveal critical trends and correlations, helping organizations craft strategies that resonate with their audience. However, questions must be designed carefully to minimize bias and ensure clarity. Surveys can miss the richness of qualitative responses and often depend heavily on the respondents’ willingness to provide honest feedback.
Statistical Modeling
Statistical modeling refers to the use of mathematical models to represent data relationships, facilitating predictions and insights into trends. This method is highly regarded in various fields, from finance to health care, where understanding the likelihood of outcomes can lead to better decision-making. The ability to build models that accurately reflect variables at play is not just beneficial but essential in navigating complexities.
The unique feature of statistical modeling lies in its predictive power, allowing organizations to forecast future events based on historic and current data. However, these models can be influenced by underlying assumptions, which, if incorrect, can lead to misguided conclusions. So while beneficial, caution is necessary.
Regression Analysis
Regression analysis is a vital statistical method used for investigating the relationships between variables. It allows analysts to determine the degree to which one variable influences another, creating a clear picture of how various factors interact. The central advantage of regression analysis is its ability to control for multiple variables simultaneously, revealing insights about causality rather than mere correlation.
The key characteristic of regression is its versatility. Analysts can use it in finance for predicting sales, in healthcare for assessing treatment efficacy, and in many fields where understanding influences is critical. But, like any method, it has drawbacks; for instance, it assumes that relationships are linear, which may not always hold true in real-world scenarios. Still, regression offers a robust tool for both exploration and explanation, making it indispensable in data analysis.
Applications of Data Analysis Skills
The realm of data analysis skills extends far beyond mere number crunching. These abilities have become instrumental in multiple fields, particularly business and scientific inquiry. Understanding how to effectively utilize data can fundamentally alter decision-making processes. Whether one is a seasoned executive or a fresh-faced researcher, mastering these skills can lead to more informed conclusions and strategic advantages.
Business Decision-Making
In business, data analysis serves as the backbone for making significant choices. It empowers companies to sift through the chaos of information and zero in on actionable insights that can streamline operations and enhance profitability.
Market Research and Trend Analysis
Market research and trend analysis delve into understanding customer behavior and market dynamics. This aspect forms the crux of many business strategies, as it aids in identifying gaps in the market or new customer preferences. A key characteristic of market research is its ability to paint a comprehensive portrait of potential markets and audiences.
- This method is often celebrated for its adaptability. Businesses can leverage primary data—direct from surveys and feedback—or secondary data, which includes reports and articles.
- Market research’s unique feature is its capacity to predict shifts in customer purchasing behavior, providing businesses a timely opportunity to adapt.
- Advantages include enhanced customer satisfaction and targeted marketing campaigns, while potential disadvantages might manifest as high costs or time-consuming processes.
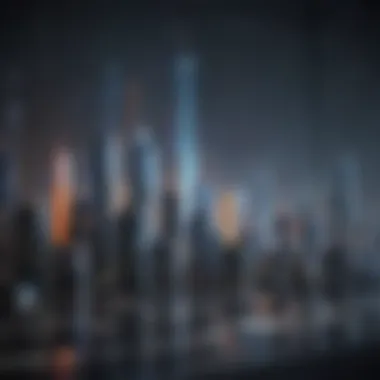
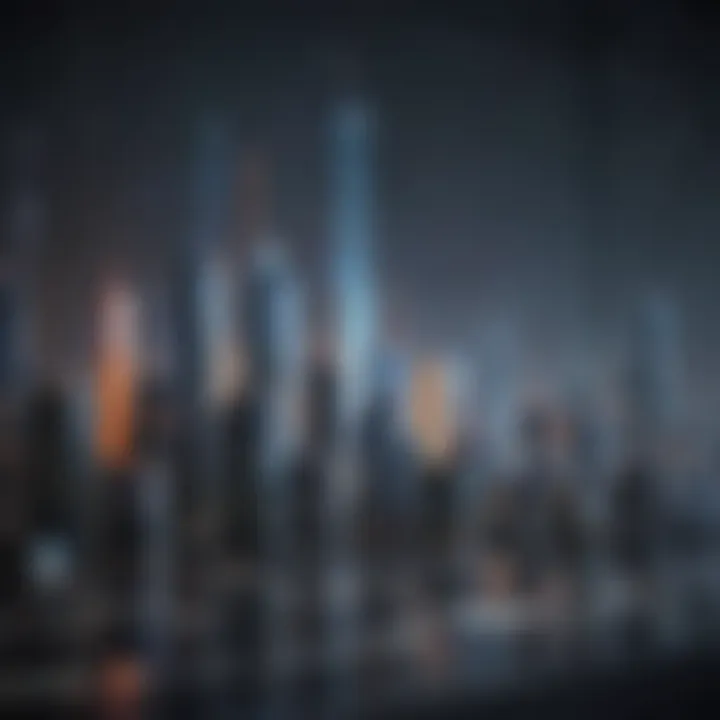
Performance Metrics Evaluation
Evaluating performance metrics is another vital facet of data analysis in business. This approach allows firms to gauge their success in various areas through measurable indicators, such as sales figures and engagement rates. Its key characteristic lies in its focus on quantifiable outcomes, making it an obvious choice for organizations striving for efficiency.
- The uniqueness of performance metrics evaluation comes from its data-dense nature, allowing organizations to quantify not just profits but also employee productivity and customer retention.
- The advantages here are clear: identifying underperforming areas can drive strategic change. However, a disadvantage could be a potential over-reliance on numerical data at the expense of qualitative insights.
Resource Allocation Strategies
Resource allocation strategies focus on the judicious distribution of an organization's assets—be it financial, human, or technical resources. This is crucial in ensuring that the right projects get the attention and funding they need to succeed. The key characteristic of this approach is its emphasis on optimizing resources to achieve maximum output.
- What sets it apart is the ability to leverage data insights for forecasting, which can lead to more effective planning and investment decisions.
- The advantages are manifold, leading to reduced waste and improved profitability. On the flip side, a disadvantage could be the complexity involved in accurately assessing all resource needs and potential returns.
Scientific Research
Transitioning to scientific research, data analysis becomes a pivotal element that underscores the rigor and reliability of studies. This process not only facilitates groundbreaking discoveries but also ensures that research findings are robust and reproducible.
Experimental Design
Experimental design is foundational for any scientific inquiry. It ensures that researchers set a standard by which the impact of various factors can be measured. The key characteristic of experimental design is its structured framework, allowing for controlled tests that can yield replicable results.
- Its unique feature lies in its emphasis on establishing control and treatment groups, which can clarify causal relationships—an essential element in scientific discourse.
- Advantages include the capacity to draw valid conclusions and generalize findings to larger populations. However, a disadvantage is the potential for biases if randomization is not effectively executed.
Data Collection and Interpretation
Data collection and interpretation are vital in the journey of scientific persistence. Accurate data collection methods ensure that research findings are credible and informative. The key characteristic here is the stringent protocols followed to gather and assess data—whether it be qualitative or quantitative.
- The unique feature of this process is its dual nature; it does not just involve gathering information but also critically interpreting that information to draw meaningful conclusions.
- Advantages include heightened accuracy in findings, whereas a significant disadvantage is the resource-intensive process that may deter comprehensive data collection.
Research Outcome Validation
Lastly, research outcome validation consolidates the integrity of scientific findings. This process is not merely a formality; it is essential for confirming the validity of research claims. The key characteristic of this process is its reliance on replication and peer review to ensure results stand up against scrutiny.
- What makes it unique is its systematic approach in forming a consensus among the scientific community, leading to accepted knowledge.
- The advantages here are undeniable, as they enhance the credibility of research. Conversely, a potential disadvantage comes to light when the validation process hinders timely dissemination of findings, as lengthy reviews can delay publication.
In summary, the applications of data analysis skills reveal their profound impact, reshaping decision-making in both business and scientific domains. Their importance cannot be overstated, as organizations and researchers alike depend on these skills to navigate an increasingly complex landscape.
The Evolution of Data Analysis Skills
The landscape of data analysis skills has evolved dramatically, influenced heavily by technological advancements. Understanding this evolution can shed light on how these skills have transitioned from basic statistical methods to sophisticated analytical techniques, which now often incorporate both big data and machine learning. This evolution is not just a chronological narrative but a reflection of changing needs across various sectors including business, healthcare, and academia. It underlines essential elements for anyone keen on adapting to the ever-changing data-driven world.
Technological Advancements Impacting Data Analysis
Emergence of Big Data
The emergence of big data marks a significant milestone in the data analysis sphere. It refers to the vast volumes of data that exceed the capabilities of traditional data processing applications. The specific aspect worth noting here is how big data allows organizations to access a wealth of information that was previously inaccessible. This creates opportunities for deeper insights and more informed decision-making processes.
One key characteristic of big data is its high velocity, which means data is generated and needs to be analyzed in real-time. This ability to analyze streams of data on the fly is enticing for businesses aiming to stay ahead of the curve. However, alongside its advantages—like enhanced insights and predictive analytics—big data also brings challenges such as data governance and quality issues, which can complicate the analytical process.
Machine Learning Integration
Another game changer is the integration of machine learning into data analysis. This technology enables systems to learn and adapt from the data without explicit programming. It allows for the construction of models that can predict future trends based on historical data. The specificity here revolves around its capability to improve accuracy in analyses over time.
The key characteristic of machine learning is its ability to handle complex datasets far more effectively than classical statistical methods. It has become a popular choice for analysts looking to automate processes and uncover hidden patterns. However, it is important to note that reliance on machine learning models can also create a black box effect, making it harder to interpret results.
Cloud Computing Solutions
Cloud computing solutions represent another leap forward in data analysis capabilities. The specific aspect that deserves attention is the scalability it offers. Organizations can run complex analyses without investing heavily in physical infrastructure. This flexibility enables companies to scale their data analysis efforts in line with their growth.
A key characteristic of cloud computing is its availability, providing access to analytical tools from virtually anywhere with an internet connection. This democratizes access to data analysis tools, making them more widely available across different sectors. However, while cloud solutions come with numerous benefits, they may also face challenges related to security and data privacy, which are becoming central considerations in today’s context.
Future Trends in Data Analysis Skills
Data Ethics and Privacy Considerations
As data analysis becomes more prominent, data ethics and privacy considerations become critical. The specific aspect here involves regulatory frameworks that govern data use. Understanding these regulations is paramount for analysts seeking to operate within legal bounds while retaining customer trust.
The key characteristic of data ethics is its focus on responsibility in data handling. This ongoing dialogue about ethics is not only beneficial but necessary as it fosters responsible analytics practices. Unique to this aspect is the emphasis on transparency, ensuring that stakeholders are informed about how their data is used.
Augmented Analytics
Augmented analytics is another trend that’s shaping the future of data analysis skills. This technology simplifies data preparation and enables automated insights, making it a crucial element for enhancing data literacy among non-specialists. The notion here is that it lowers the barrier to entry for those who may not be professionally trained in data analysis.
A key characteristic of augmented analytics is its user-friendliness, allowing individuals with varied backgrounds to engage with data meaningfully. However, one disadvantage lies in the potential for over-reliance on automated systems, which could diminish critical thinking and analytical skills among users.
Increasing Demand for Data Savvy Professionals
Finally, there’s an increasing demand for data-savvy professionals. This reflects a broader industry recognition of the value that skilled data analysts bring to organizations. The specific aspect worth discussing is the growing emphasis on continuous learning and upskilling in this area.
The key characteristic here is the integration of data skills across disciplines; it’s no longer just the domain of data professionals. Diverse fields such as marketing, finance, and healthcare are recognizing the need for professionals who can interpret data effectively. A unique feature of this trend is the rise of interdisciplinary teams, combining skills from various sectors. However, the potential drawback is the risk of becoming overwhelmed with the sheer volume of tools and techniques available, making it essential for professionals to focus on what fits their specific needs.
As the data analysis landscape changes, so too must the competencies associated with it, calling for professionals who are not only data proficient but also ethically aware and technologically savvy.