Top Players in AI Chip Manufacturing Industry
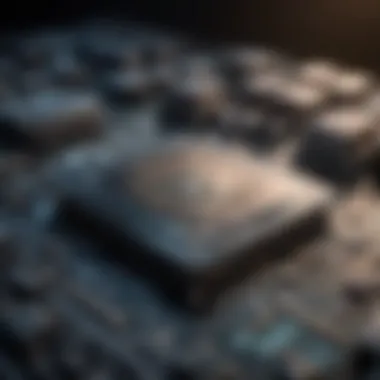
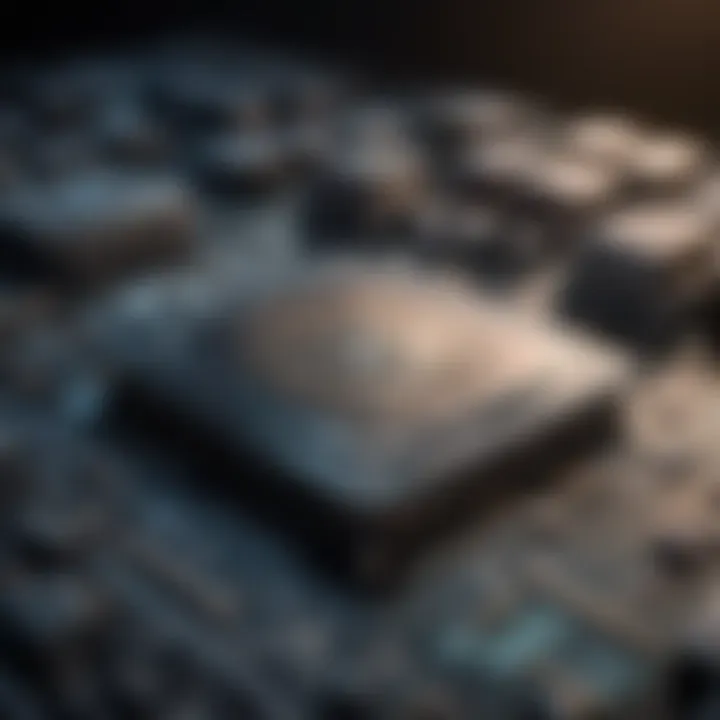
Intro
Artificial Intelligence (AI) chips represent the backbone of the rapidly advancing field of artificial intelligence, powerfully influencing industries ranging from healthcare to autonomous vehicles. As we stand on the precipice of an AI-driven era, understanding the key players in the AI chip manufacturing sector becomes crucial. This exploration lends clarity on not only who is leading the pack but also how their innovations are shaping the technology landscape.
By the end of this article, the reader will have a thorough understanding of the leading companies in AI chip manufacturing. We will discuss their strategies, technological advancements, and the broader implications of their contributions within a competitive market. In this fast-paced environment, staying updated on these developments matters not just for tech enthusiasts but also for investors looking to navigate this dynamic sector with insight.
Key Concepts and Definitions
Before diving into the intricate details about the companies at the forefront of AI chip manufacturing, it’s beneficial to grasp some foundational terms and concepts in this domain.
Overview of Investment Terms
When discussing investments in AI chips, familiarizing oneself with specific terminologies is imperative. Here are some key terms to know:
- Market Capitalization: This refers to the total value of a company's outstanding shares, providing insight into the size of the company within the industry.
- R&D Investment: This term signifies the funds allocated towards research and development, crucial for innovation in AI chip technology.
- Intellectual Property (IP): Refers to the use of patents and trademarks, particularly in a field where unique technologies emerge, influencing a company’s competitive edge.
- Supply Chain Management: The process of overseeing the production flow of AI chips, ensuring quality and efficiency from materials to delivery.
Significance of Understanding Financial Terminology
Grasping these financial terms lays the groundwork for comprehending the strategic maneuvers of AI chip manufacturers. Without this context, it's easy to miss the nuances that drive market behaviors and company performance. For instance, a significant increase in R&D investment might indicate an impending breakthrough or a new product launch, thus impacting market perceptions and stock prices.
"Understanding the language of finance is akin to having the right map in a complex terrain; it guides you through the intricacies of market dynamics and competitive strategies."
With a grasp on these concepts, readers are better equipped to appreciate the analytical insights that follow. This knowledge serves not only to bolster individual understanding but also empowers informed discussions around investments in technology—a domain that’s ever-changing and full of potential.
Prolusion to AI Chip Technology
In today’s digital environment, AI chip technology emerges as the very heart of numerous innovations. The relevance of this topic is underscored by its profound impact on how we perceive and utilize artificial intelligence across various sectors. AI chips are specially designed to handle the intricate algorithms and processes involved in machine learning, making them pivotal for enhancing computing power and efficiency.
Definition and Purpose of AI Chips
AI chips, at their core, are semiconductor devices tailored to perform AI-related tasks. They are fundamental in accelerating several operations, from data processing to neural network computations. The purpose of these chips goes beyond mere speed; it's about optimizing for specific workloads. For instance, rather than using a general-purpose CPU, companies opt for AI chips because they can handle parallel processing tasks effectively. This allows technology firms to deliver smoother experiences in applications like image recognition or natural language processing. In today’s market, where every millisecond counts, the efficiency provided by AI chips is a game-changer.
The Evolution of AI Chips
The journey of AI chips is nothing short of remarkable. Initially, the notion of artificial intelligence was dominated by general-purpose CPUs, but the landscape shifted in the late 2000s with the burgeoning emphasis on parallel processing. Early on, graphics processing units (GPUs) started getting recognition, as they could process multiple calculations simultaneously, making them suitable for training deep learning models.
However, it was shortly followed by a wave of specialized chips designed exclusively for AI tasks. Companies recognized that these new, specialized processors could provide orders of magnitude better performance compared to their predecessors.
- In recent years, we’ve seen a flurry of developments with the introduction of Tensor Processing Units (TPUs) by Google, providing another avenue for enhanced computation.
- Simultaneously, other companies aimed to push the envelope with innovative architectures and designs focused solely on AI workloads.
This evolutionary path highlights not only technological advancements but also the growing demand for efficient processing power in a world increasingly driven by data. As businesses and developers look to harness the potential of AI, the evolution of these chips remains at the forefront, offering new solutions and enhancing capabilities.
The Importance of AI Chips in Modern Computing
Artificial intelligence chips are not just another piece of hardware; they are the lifeblood of today's tech-driven landscape. Their significance lies in the remarkable abilities they bring to systems, allowing unprecedented processing power, efficiency, and speed. As industries pivot toward ever more complex algorithms and massive datasets, the integration of AI chips becomes essential for scaling operations and improving service delivery.
Applications Across Various Industries
AI chips find applications all across diverse industries, each utilizing their unique capabilities to address specific needs and challenges. Here’s a closer look at a few key sectors:
Healthcare
In the healthcare sector, AI chips play a crucial role in the rapid processing of medical data. From imaging diagnostics to predicting patient outcomes, these chips enhance the accuracy and speed of analysis, leading to quicker interventions. A key characteristic of AI in healthcare is its ability to analyze vast quantities of data from various sources, such as electronic health records and genetic sequences. This ability makes it a popular choice for improving patient care.
The unique feature here is the chips' capacity to perform real-time analytics, which can significantly reduce the time doctors spend on diagnostics. However, there can be disadvantages—such as concerns about data privacy and the potential for biases in algorithms that lead to misdiagnoses.
Automotive
In the automotive industry, AI chips are pivotal in the development of autonomous driving technologies. These chips process data from myriad sensors and cameras, enabling vehicles to make split-second decisions. A standout aspect of automotive AI is the chips' integration with machine learning, which continuously improves the vehicle's performance based on driving conditions.
The unique feature is their ability to learn from real-world scenarios without human intervention. Yet, the challenge remains in the high stakes of safety and reliability that the technology demands—any malfunction could lead to catastrophic consequences.
Finance
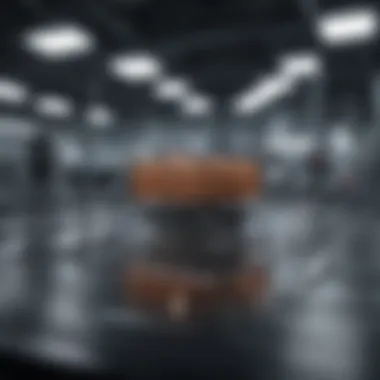
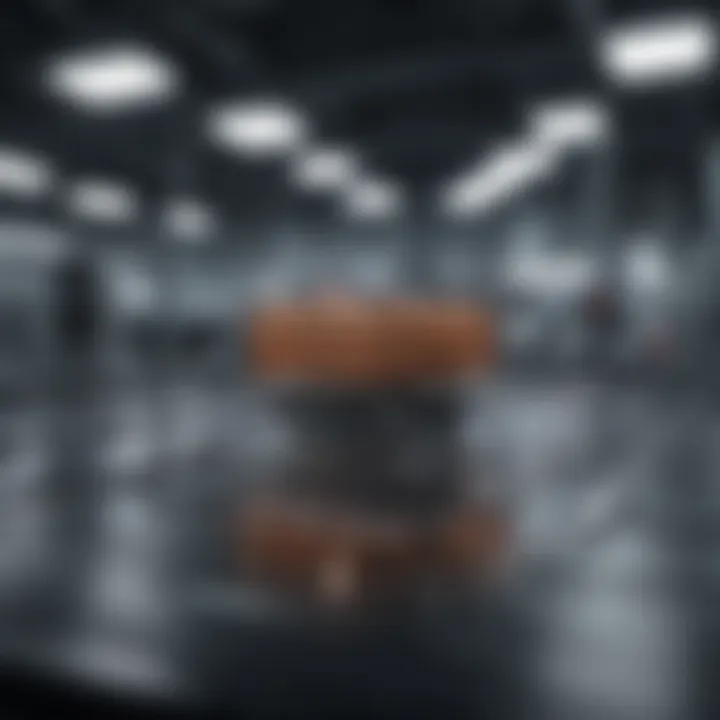
In finance, AI chips are transforming trading strategies and risk assessment models. They facilitate the advanced algorithms that analyze market trends, enabling faster decision-making than ever before. The distinguishing characteristic of AI in finance lies in the capacity to process large datasets in real time, providing traders with actionable insights almost instantaneously.
A unique aspect is their role in automating routine tasks, freeing up human analysts to focus on strategic decisions. While this offers significant advantages, such as minimizing human error, it also raises concerns about the market volatility driven by algorithmic trading.
Robotics
The robotics field heavily relies on AI chips for tasks such as navigation and object recognition. Here, the chips allow robots to interpret their environment and make decisions autonomously. This application stands out due to the versatility and adaptability of AI in various robotic systems, from manufacturing to personal assistants.
The groundbreaking aspect is the ability for these robots to learn from their experiences over time. However, there are disadvantages too; the complexity of programming and the integration of AI can pose significant hurdles, not to mention the ethical implications of autonomous machines in the workplace.
Impact on Efficiency and Performance
The efficiency and performance of systems enhanced by AI chips cannot be overstated. These chips streamline processes, allowing tasks that would have taken humans hours to complete to be done in a matter of seconds. They manage and process information with a remarkable speed that traditional chips can’t match.
“AI chips are redefining performance benchmarks, allowing businesses to operate at unprecedented levels of efficiency.”
One of the core benefits is the reduction in power consumption while maintaining high performance, which is critical as industries increasingly prioritize sustainability.
Overall, the importance of AI chips in modern computing is evident across various sectors, with their ability to transform processes and make previously impossible tasks feasible.
Key Players in the AI Chip Market
In an era where technology evolves at the speed of light, understanding the key players in the AI chip market is crucial. These companies aren't just manufacturers; they shape the landscape of artificial intelligence, influencing how we interact with technology daily. Their advancements in chip technology directly affect various sectors including healthcare, automotive, and finance. The decisions these firms make ripple through the industry, making awareness of their strategies vital for investors and tech enthusiasts alike.
One of the unique aspects of these market leaders is their ability to innovate while maintaining a competitive edge. Their contributions help streamline processes and enhance performance across sectors, creating a synergy that benefits all. Investing time to study these companies can provide insight not just for market potential, but also for the direction technology is heading. Let's delve into some of the most influential players in this market and understand what makes them stand out.
NVIDIA: Pioneering AI Graphics Processing
Technological Innovations
NVIDIA has made a name for itself with its groundbreaking technological innovations, specifically in the realm of graphics processing. The company's GPUs are not just about rendering graphics; they’re designed to handle the demands of deep learning and neural networks. This shift to AI-centric processing puts NVIDIA at the forefront of the AI chip market.
Adopting their GPUs means leveraging immense parallel processing capabilities, which allows businesses to conduct complex computations in a fraction of the usual time. This is a game changer in fields, from autonomous vehicles to medical imaging. Their recent developments like Tensor Cores enhance performance specifically for AI tasks, making it a popular choice in this article due to efficiency and speed in tasks.
Market Position
NVIDIA's market position is something to marvel at. With a dominant share, they consistently outshine rivals in the GPU space. The strategic move towards AI has solidified their place, allowing them to cater to a growing demand for AI computing power. Their brand recognition is intertwined with high performance, often being the go-to for tech enthusiasts.
However, this positioning also brings challenges. As more competitors enter the fray, NVIDIA must innovate constantly to maintain its stature. Their flagship products have unique features that blend advanced technology with user-friendliness, but market dynamics can be quite ruthless.
Intel: Diversifying AI Solutions
Acquisitions and Partnerships
Intel has taken a multi-faceted approach by diversifying its AI solutions through timely acquisitions and strategic partnerships. By acquiring companies like Nervana Systems, Intel not only boosts its technological capabilities but also broadens its competency in AI chip manufacturing. This strategy facilitates access to innovative technologies that can be integrated with existing systems, which is essential for keeping pace with rapid developments in AI. Adapting this approach allows Intel to respond quickly to changing market demands, ensuring their products are relevant and cutting-edge. Not to mention, partnerships with firms in industries such as automotive and healthcare allow for cross-industry collaborations, creating a synergy that benefits all parties.
Product Development
When it comes to product development, Intel stands tall. Known for its x86 architecture, the company not only caters to traditional computing needs but is now stepping into the AI domain actively. Their focus on developing specialized processors like the Intel Nervana Neural Network Processor underscores this shift. This is strategically significant, as it positions Intel as a serious contender in the AI chip space.
Nevertheless, they face a double-edged sword. Their immense product line may sometimes lead to confusion among consumers about which chip suits their specific needs. Keeping products user-friendly while catering to advanced users is a delicate balance.
AMD: Competing with Performance
Product Line overview
AMD has carved out its niche by concentrating on performance and efficiency in its product line. Their Ryzen and EPYC processors have gained traction due to their multi-core capabilities, making them ideal for AI workloads. This is especially beneficial for sectors focusing on data-intensive processes. With each launch, AMD enhances its offerings, pushing for better performance metrics to match or exceed competitors' specifications.
The unique aspect of AMD's product line is its balance between power and price, providing incredible value to consumers and businesses alike. However, they must still contend with NVIDIA’s established dominance in the GPU space.
Market Strategy
AMD's market strategy is noteworthy for its focus on aggressive pricing tactics and performance benchmarks. By offering chips that deliver on both fronts, they attract not just gamers and tech enthusiasts but also enterprise-level clients. This is a beneficial route as Intel traditionally commanded a higher price premium.
Their strategy does come with risks, particularly in supply chain management and production consistency. High demand can lead to shortages, forcing customers to look elsewhere and potentially eroding trust in their reliability.
Google: Innovating with TPUs
Custom Chip Development
Google dives into custom chip development like no other with its Tensor Processing Units (TPUs). These specialized chips are designed specifically for machine learning tasks, showing remarkable efficiency in training AI models. By creating its chips, Google gains substantial control over hardware and software synergy, enhancing overall performance.
The unique feature of these TPUs is their ability to handle large-scale computations faster than traditional chips, making them a valuable asset in Google's data center operations. However, this means they might not appeal broadly outside Google's ecosystem, as they are tailored for specific tasks.
Cloud Applications
Google's cloud applications are revolutionizing the way industries utilize AI. With offerings such as Google Cloud AI and BigQuery, customers can leverage Google’s TPUs to run their AI models without needing in-depth hardware knowledge. This lowers the barrier to entry for companies wanting to adopt AI solutions, making it accessible even for small enterprises.
The partnership with their cloud services offers powerful computational capabilities but also raises questions about data security and privacy. Users need assurance that their data is safe while taking advantage of the computational prowess.


Apple: Customization in AI Processing
SoC Developments
Apple's System on Chip (SoC) developments reflect a serious commitment to integrating AI processing seamlessly across its devices. The M1 chip, for example, showcases how Apple is focusing on efficiency without compromising performance. This chip incorporates a neural engine specifically designated for machine learning tasks, which gives Apple a significant advantage in mobile AI processing.
What sets this apart is how it allows for real-time data processing while maintaining low power consumption, a key feature for mobile devices. However, developers seeking to optimize apps exclusively for Apple’s hardware might face a steep learning curve.
Integration Across Devices
One of Apple’s standout features is its commitment to integration across devices. The chips they develop allow for a user experience that’s smooth and cohesive, whether on an iPhone, iPad, or MacBook. These devices share processing capabilities, allowing for seamless transitions between tasks, especially for AI applications like image recognition or personalized recommendations.
It’s a beneficial strategy, but it does limit compatibility with third-party software and may frustrate users accustomed to broader ecosystems. The challenge remains in balancing innovation while not alienating potential customers.
Emerging AI Chip Companies of Note
With the pace of AI chip development accelerating, a wave of emerging companies is challenging established players in the industry. These newcomers are not just fitting into the existing framework but are also reshaping how we view artificial intelligence's hardware landscape. Their unique offerings can address specific needs in the market, especially as AI applications become more widespread and complex.
Emerging companies play a pivotal role in innovating and enhancing AI chip technology, often finding niches that larger enterprises may overlook. This can lead to significant advancements that foster more efficient computing experiences across various sectors. Veterans like NVIDIA and Intel established the groundwork, but companies such as Graphcore, Cerebras Systems, and SambaNova Systems are rising to the occasion. These firms focus on specific aspects of AI processing, which is essential in a crowded field.
Investors and tech enthusiasts should pay attention to this dynamic segment in the industry. The growing prominence of these companies offers opportunities not only for capital but also for advancements in technology that can revolutionize sectors like healthcare, automotive, and beyond.
Graphcore: Revolutionizing AI with IPUs
Graphcore has made quite a stir within the AI chip arena with its Intelligence Processing Units (IPUs). These specialized chips are designed specifically for the unique requirements of AI workloads, offering enhanced performance compared to traditional processors. Neuromorphic computing, which imitates human brain activity, underpins their development, allowing for parallel processing and faster execution of machine learning tasks.
Graphcore’s strategic focus on developing IPUs enables organizations to train models with greater efficiency. As AI models grow in complexity, the need for optimized hardware becomes all the more critical. IPUs offer a roster of advantages:
- Higher throughput for machine learning tasks.
- Scalability to accommodate different AI workloads.
- Efficiency that reduces power consumption, beneficial for large data centers.
Their partnerships with major cloud providers like Microsoft Azure further demonstrate Graphcore's commitment and growing relevance in the market.
Cerebras Systems: Giant Chips for High-Performance Computing
Cerebras Systems takes a bold approach by creating exceptionally large chips, exemplifying innovation in the AI chip manufacturing realm. Their flagship product, the Wafer Scale Engine, occupies 46,225 square millimeters, making it one of the largest chips ever manufactured. This colossal size allows for vast numbers of processing cores, delivering immense computational power necessary for deep learning and complex calculations.
The key advantages provided by Cerebras's technology include:
- Extreme parallelization, enabling efficient handling of extensive datasets.
- Exceeding the memory bandwidth of traditional GPU solutions, which is crucial for AI workloads.
- Simplified infrastructure for large-scale AI models, reducing the number of chips and connections needed.
Through these features, Cerebras Systems positions itself as a game-changer, especially in research environments that demand high-performance computing.
SambaNova Systems: Enabling AI for Enterprises
SambaNova Systems is carving its niche by offering enterprise solutions tailored for AI development. This company is focused extensively on building advanced systems that allow businesses to leverage AI capabilities seamlessly. SambaNova's architecture promotes flexibility, enabling enterprises to execute machine learning workloads efficiently without a massive investment in infrastructure.
Their offerings emphasize:
- Streamlined AI deployment, simplifying how organizations utilize AI technology.
- Integration of hardware and software to create an optimal environment for deep learning applications.
- Security and compliance features that are essential for companies dealing with sensitive data.
By taking a comprehensive approach, SambaNova Systems is making it easier for enterprises to adopt AI solutions, thus boosting productivity and paving the way for future innovations.
These emerging companies illustrate how the AI chip sector continues to evolve, laying the foundation for how technology will shape various industries and enhancing the possibilities of what AI can achieve.
Technological Advancements Driving AI Chip Development
The realm of AI chip development stands at the intersection of relentless innovation and emergent needs across industries. As we delve into the technological advancements that are reshaping this landscape, it becomes clear that these innovations are not mere enhancements but essential drivers of progress within the sector. This section elucidates how novel manufacturing techniques and the architecture of chips are paving the way for more powerful and efficient AI solutions.
Manufacturing Techniques and Materials
Semiconductor Innovations
Semiconductor innovations are undoubtedly the backbone of modern AI chip technology. They introduce a plethora of materials that enhance chip performance while reducing energy consumption. A key characteristic of these innovations is the shift towards advanced materials such as Gallium Nitride and Graphene. These materials not only offer superior conductivity compared to traditional silicon but also facilitate higher operational temperatures and efficiencies.
One unique feature of these semiconductor innovations is their scalability. This scalability allows manufacturers to produce chips in larger quantities without sacrificing performance. It’s a popular choice for companies looking to cut down costs while still pushing the envelope in terms of computational power. However, it’s noteworthy to mention that the complexities involved in integrating these materials can present challenges. Manufacturers often face hurdles with heat management and reliability, which can deter full-scale deployment.
Chip Architecture
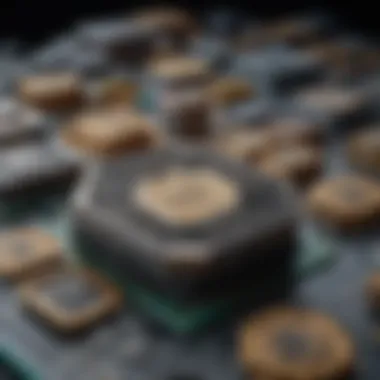
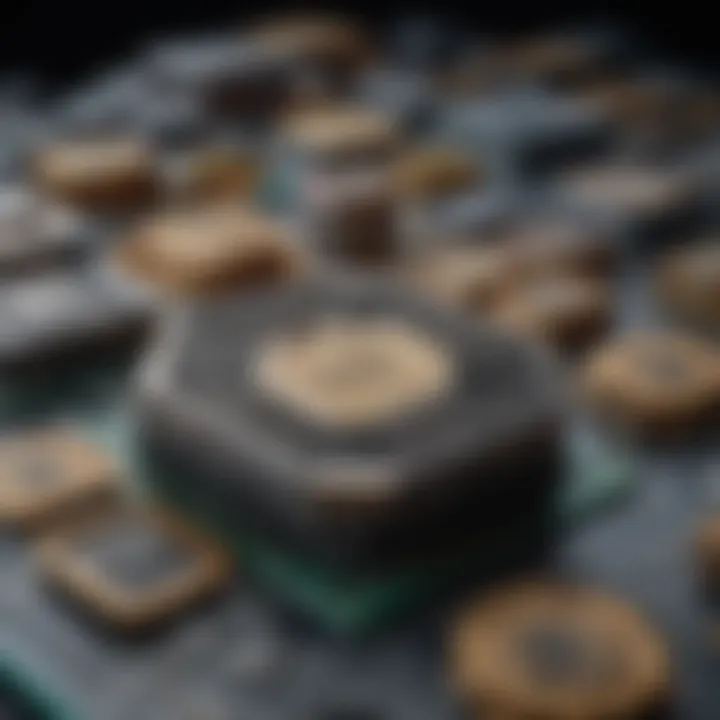
When considering chip architecture, we see an evolution driven by the need for parallel processing—crucial for AI applications that require massive data handling. A prominent trend is the shift towards modular designs. This design approach enables customization for various applications and significantly increases processing efficiency. The ability to tailor architecture can lead to reductions in latency, making it a favorable choice for real-time applications.
A unique aspect of modern chip architecture is its focus on energy efficiency alongside performance. Chips are being designed to minimize power consumption while maximizing output, a necessity given the rising costs of energy globally. But with benefits come considerations; the complexity of these architectures can lead to longer design cycles, which might delay product availability in fast-paced markets.
Software Optimization for AI Chips
The next piece of the puzzle lies in software optimization. In this space, it is not just about having cutting-edge hardware; the synergy between software and AI chips is paramount. Optimized software can drastically enhance chip performance, squeezing every last drop of efficiency from the silicon.
Currently, there are several frameworks that aim to bridge the gap between hardware capabilities and software demands. Popular ones include TensorFlow and PyTorch, which enable developers to efficiently run complex algorithms on AI chips. However, software optimization is an ongoing process, needing constant updates to align with hardware advancements. It requires collaboration between software engineers and chip designers—an intricate dance of sorts—to ensure compatibility and performance.
Ultimately, technological advancements are steering the AI chip industry towards more sophisticated and effective solutions. As we stand on the brink of a revolution in AI capabilities, the interplay between manufacturing techniques, chip architecture, and software optimization will be critical. Ensuring that these elements work harmoniously can lead to the next groundbreaking AI applications, reshaping industries from healthcare to finance.
"The landscape of AI chips is nothing short of a chess game, where each move in technology paves the way for your next big leap."
In this context, understanding these advancements not only helps one appreciate the present state of AI chip technology but also prepares us for the innovations that lie just around the corner.
Challenges in the AI Chip Industry
The landscape of AI chip manufacturing is not without its hurdles. While the companies leading the charge in this sector demonstrate remarkable innovation and strategic foresight, they also contend with significant challenges that could impact their trajectories. Understanding these issues helps frame the broader context of the industry and highlights the resilience required by companies to navigate an increasingly complex global environment.
Supply Chain Constraints
One of the most pressing issues in the AI chip market is supply chain constraints. These constraints stem from various factors, including the ongoing global semiconductor shortage, which has affected many industries beyond just technology. Companies often face difficulties in sourcing raw materials, which can lead to delays in production and increased costs.
For instance, the shortage of essential components like silicon wafers has forced some manufacturers to rethink their supply strategies. With demand outpacing supply, companies find themselves in a tight spot, scrambling to secure enough resources to meet their production quotas. This not only hampers growth but can also impact their competitive edge in the market.
The ripple effect of these constraints is significant. It affects planning and procurement processes, causing companies to adopt just-in-case inventories rather than just-in-time models. This means that cash flow management becomes a balancing act, and companies may see their profit margins squeezed as they invest more into managing supply risks.
Intense Market Competition
Market competition in the AI chip sector is fierce and relentless. With a growing number of players entering the field, established companies face the threat of new entrants armed with innovative technologies that could disrupt the status quo. This competitive pressure encourages constant innovation, but it can also lead to price wars that can be detrimental to profit margins.
Moreover, established giants like NVIDIA and Intel must also fend off emerging players like Graphcore and Cerebras Systems, who are pushing the envelope with unique architectures and design philosophies. The aggressive pursuit of market share necessitates not only high-quality products but also a robust marketing strategy to carve out a niche in the crowded marketplace.
"Competing effectively means being agile – adapting to market changes faster than the competition. Firms cannot rest on their laurels; stagnation can be as harmful as a lack of innovation."
This environment of intense competition forces firms to continuously invest in research and development. Allocating funds to innovation, while maintaining operational efficiency, becomes a challenging psychological and economic gamble. Just think about it: companies that rest too long on their current technologies may find themselves outpaced and irrelevant as the market evolves.
Regulatory Factors and Global Trade
Regulatory challenges are another significant hurdle in the AI chip industry. Companies must navigate a complex web of regulations that vary by country and region, typically concerning data protection, trade laws, and intellectual property rights. For instance, international trade disputes can restrict supply chains, forcing companies to find alternative routes or sources, often at a higher cost.
Furthermore, regulatory compliance can slow down the pace of innovation. Companies often need to allocate resources to ensure their products meet the requisite standards, which can extend timelines for new chip releases. A clear example of this is the scrutiny surrounding data privacy regulations and their implications for AI technology, compelling makers to fundamentally alter their approaches.
In a globalized market, companies must be aware of geopolitical dynamics that can impact trade relations. Changes in tariffs, export controls, or bans can hinder a company’s ability to sell their products in certain markets or spark retaliatory measures, leading to abrupt shifts in strategy.
Navigating these regulatory waters requires not only awareness but also the ability to adapt promptly, as sometimes, the rules of the game can change overnight. Being nimble and proactive is essential for AI chip manufacturers in this climate, as they balance compliance with the need for innovation and growth.
Future Outlook of AI Chip Manufacturing
As the demand for artificial intelligence capabilities skyrockets, the future of AI chip manufacturing appears both exciting and crucial. With various industries leaning heavily on AI for improved efficiencies and insights, the evolution of AI chips promises numerous benefits, but it also poses some considerations that need addressing. Understanding these dynamics is vital for stakeholders, including investors and tech enthusiasts, who seek to grasp how the market might unfold in the coming years.
With advancements in technology, companies are increasingly investing in AI chips to facilitate deep learning and enhance processing speed. Thus, market predictions suggest that the growth rate for AI chip manufacturing can reach unprecedented levels. Companies are focusing on optimizing their operations and improving cost-effectiveness, ensuring sustainability in the long run.
Predictions for Market Growth
The AI chip market's trajectory looks robust. Analysts anticipate that the global AI semiconductor market will reach staggering numbers by the end of the decade. One thought-provoking analysis predicts a market growth to over $200 billion by 2030, driven by demand from sectors such as healthcare, automotive, and cloud services.
- Key reasons behind this growth:
- Increased Adoption of AI Technologies: Industries are actively integrating AI into their operations, driving demand for specialized chips that cater to such needs.
- Expansion of Edge Computing: As more devices are connected, the need for chips that can perform computations near the data source is rising. This shift is fundamental in lowering latency and enhancing performance.
- Government Investments: With advancing technologies, government agencies across the globe are investing in AI. Support through funding and initiatives can propel the growth of AI chip manufacturers.
"AI chips may become as integral to computing as standard processors have been, paving the way for revolutionary applications across sectors."
Potential Innovations on the Horizon
As for potential innovations on the horizon, we can expect some fascinating developments that could change the landscape of AI chip manufacturing. Here are a few noteworthy trends:
- Neuromorphic Chips: These are modeled after the human brain, enabling more efficient data processing and energy consumption. Companies are researching the implications of these chips for complex AI tasks, and a breakthrough in this area could lead to unprecedented advancements.
- Quantum Computing Integration: Combining AI chips with quantum computing could significantly enhance processing power. This fusion may allow for faster computations and better handling of large datasets, which is vital for deep learning and advanced algorithms.
- 3D Chip Technology: Manufacturers are exploring three-dimensional chip design, which could stack multiple layers of processing cores. This method could revolutionize how chips are developed, increasing performance without needing a larger footprint.
Innovations like these are not merely hypothetical; they are actively being pursued by leading players in the market. Staying abreast of such advancements can inform investment decisions and strategic planning in a notoriously competitive field.